TSUKUBA, Japan, Dec 2, 2024 - (ACN Newswire) - Polymers, such as plastics, are essential in many aspects of life and industry, from packaging and cars to medical devices and optic fibres. Their value comes from diverse properties that are largely determined by their monomers – the single chemical units – that make up a polymer. Unfortunately, it can be challenging to control the chemical behaviour of monomers during manufacture to achieve a desired outcome.
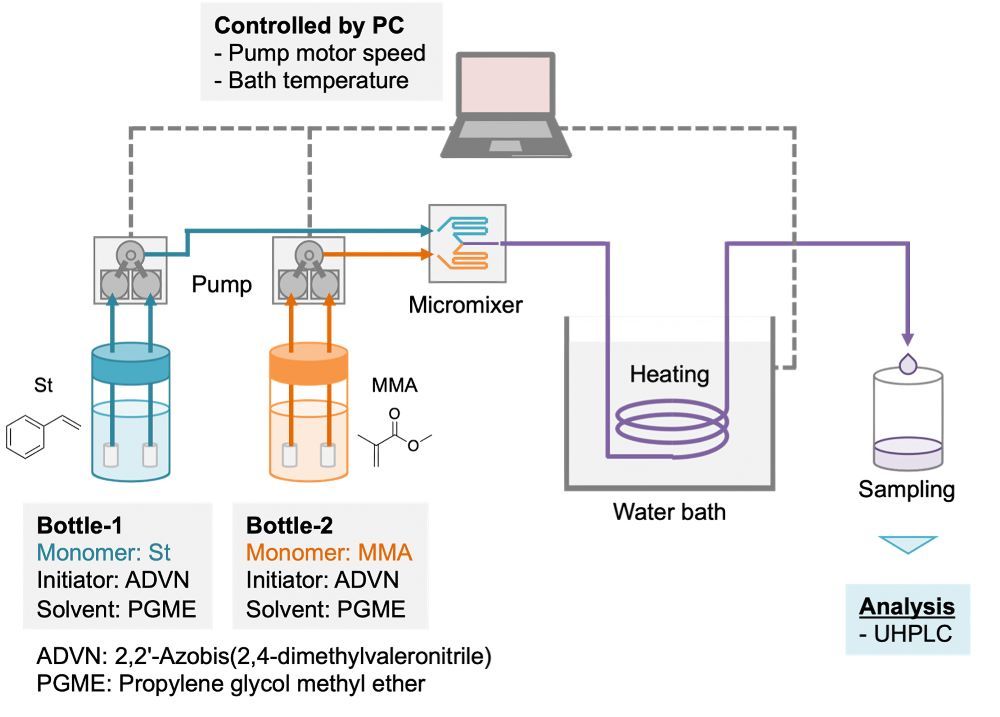
Now, a team of researchers led by Professor Mikiya Fujii of the Nara Institute of Science and Technology in Japan have used machine learning to mathematically model the polymerization process and reduce the need for time-consuming and expensive experimentation. Their results have been published in the journal Science and Technology of Advanced Materials: Methods.
Machine learning algorithms need data, so the researchers designed a polymerization process that would quickly and efficiently generate experimental data to feed into the mathematical model. The target molecule was a styrene-methyl methacrylate co-polymer, which was made by mixing styrene and methyl methacrylate monomers, both already dissolved in a solvent with an added initiator substance, then heating them in a water bath.
The team also used a method called flow synthesis, in which the two monomer solutions are mixed and heated in a constant flow. This allows for better mixing, more efficient heating, and more precise control of heating time and flow rate, which makes it ideal for use with machine learning.
The modelling evaluated the effect of five key variables in the polymerization process: the concentration of the initiator, the ratio of solvent to monomer, the proportion of styrene, the temperature of the reaction, and the time spent in the water bath. The goal was to have an end product with as close to 50% styrene as possible.
Once enough experimental data was available, the machine learning process took only five cycles of calculation to achieve the ideal proportion of styrene to methyl methacrylate. The results showed that the key was a lower temperature and longer time in the water bath, as well as lowering the relative concentration of the monomer in the solvent. The researchers were surprised to discover that the solvent concentration was just as important as the proportion of monomers going into the mix.
“Our results demonstrate that machine learning not only can explicitly reveal what humans may have implicitly taken for granted but can also provide new insights that weren’t recognized before,” Professor Mikiya Fujii says. “The use of machine learning in chemistry could open the door for smarter, greener manufacturing processes with reduced waste and energy consumption.”
Further information
Mikiya Fujii
Nara Institute of Science and Technology
Email: fujii.mikiya@ms.naist.jp
Paper: https://doi.org/10.1080/27660400.2024.2425178
About Science and Technology of Advanced Materials: Methods (STAM-M)
STAM Methods is an open access sister journal of Science and Technology of Advanced Materials (STAM), and focuses on emergent methods and tools for improving and/or accelerating materials developments, such as methodology, apparatus, instrumentation, modeling, high-through put data collection, materials/process informatics, databases, and programming. https://www.tandfonline.com/STAM-M
Dr Yasufumi Nakamichi
STAM Publishing Director
Email: NAKAMICHI.Yasufumi@nims.go.jp
Press release distributed by Asia Research News for Science and Technology of Advanced Materials.
Copyright 2024 ACN Newswire. All rights reserved. www.acnnewswire.com